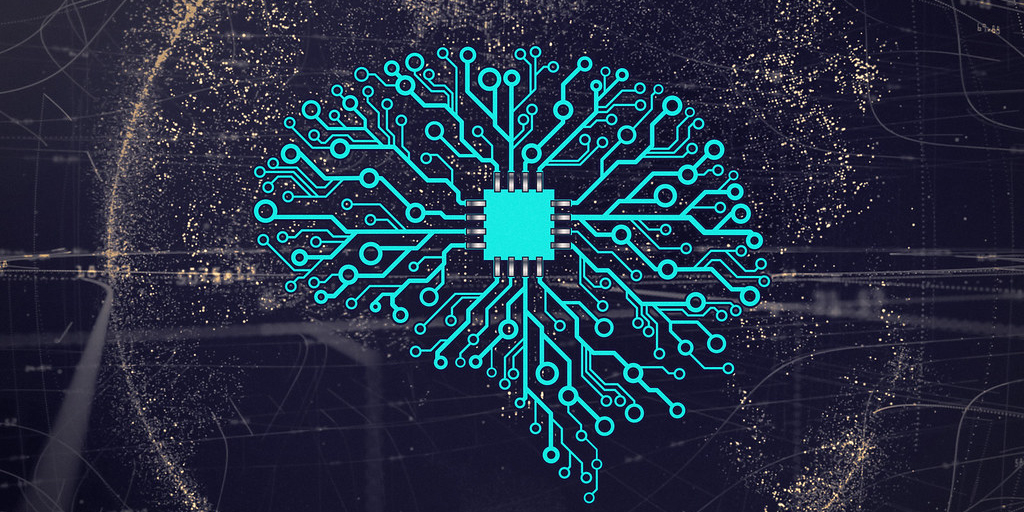
Imagining Human-AI Memory Symbiosis
By Prof. Victoria Grace Richardson-Walden, Director, Landecker Digital Memory Lab
The editors of a recent journal special issue asked, ‘Is AI the Future of Collective Memory?’. Our Director and co-author Mykola Makhortykh were invited to answer this poignant question.
The Problem of Anthropomorphism
At the heart of our contribution to the special issue was an interrogation of the problem of anthropomorphism of AI. That is – the problem of describing AI using terms related to human activity to the extent that we tend to think about it as like us or even potentially better at doing human things than us.
In the tech industry and in academic fields concerned with AI development, the narrative of artificial general intelligence (or ‘super’ intelligence) based on but one day superseding human cognitive capabilities served as a useful myth through which to market models and to attract vast levels of funding.
This challenge has not simply come from critical thinkers in the humanities and sceptics, but from AI pioneers themselves, notably Nils Nilsson and Jaron Lanier.
We took the question posed to us by the special issue’s editors Frédéric Clavert and Sarah Gensburger: ‘Is AI the Future of Collective Memory?’ and spun it on its head by focusing on re-remembering key moments in AI development to:
- really get to grips with what the specificities of AI are.
- consider potential scenarios of its use in the future for collective memory.
We took the position that we don’t really have the agency to say ‘yes’ or ‘no’ to the question posed, but rather that AI is already shaping collective memory. It is thus imperative that we interrogate it but do so through a nuanced lens focused on what it actually is, and in its own historical context, rather than blindly accepting the narratives and norms established about AI in public discourse, driven by what tech creators want users to think about it. We also wanted to avoid talking about AI in generalised terms.
We also note that this problem of anthropomorphism is also characteristic of memory studies – where we use the language of cognitive processes like remembering and forgetting to speak about media reflections on the past, and collective rituals and processes, just as we use terms like ‘intelligence’, ‘thinking’ and commands like ‘UNDERSTAND’ when developing and discussing AI.
Returning to AI Pre-Histories and Histories
There are three key works that we turn to in AI pre-history and history to demonstrate the issue of anthropomorphism and the possibility – already in these texts – of reading AI development against this grain.
The first, is Nilsson’s (2013) dense The Quest for Artificial Intelligence: A History of Ideas and Achievements in which he traces multiple pre-histories of AI, including:
- mathematics and logics from Aristotelian syllogism through Leibniz’s alphabet of human thought and calculus of reasoning, which underpin Boolean algebra (essential to algorithm design)
- Frege’s work on logic which inspired semantic networks
- and work in neuroscience, behaviourism and cognitive science which influenced the development of reinforcement learning.
In the latter particularly, AI development adopts mathematical and logical interpretations of the brain rather than taking the brain, mind, thought and consciousness on their own terms, e.g., as embodied experiences, and socio-culturally situated, affected by external stimulus as much as internal.
As such, we argue that AI development has adopted historical mathe-morphised notions of human intelligence, understanding and thinking, rather than actually trying to simulate the way these processes happen within human brains.
this is about imitation: can we be fooled enough into thinking the machine is a human player?
Simply put, the way AI developers think about the human brain is through mathematical models used to try to understand it, rather than the very messy, human nature of our actual brains and how they function and are affected by socio-cultural contexts.
We then turned to Alan Turing’s 1950 article ‘Computer Machinery and Intelligence’ in which he asked ‘can machines think?’ Whilst this question is famously attributed to him, few citations engage with what follows next in his argument, in which he quickly dismisses it as too simplistic, asking instead:
‘What will happen when a machine takes the role of A in this [imitation] game? Will the interrogator decide wrongly as often as when the game is played like this as he does when the game is played between a man and a woman?’
as he does when the game is played between a man and a woman?’
Turing goes on to demonstrate his interest is in distinguishing between what he calls ‘discrete-stage’ (e.g., computers) and ‘continuous-stage’ machines (e.g., humans), rather than whether human intelligence can be simulated.
The clue is also in the name of his experiment – this is about imitation: can we be fooled enough into thinking the machine is a human player?
As critical AI theorist Natale (2021) argues, Turing’s much misunderstood game is not the only example of the ‘illusory character of computers’ intelligence’, Joseph Weizenbaum’s ELIZA – considered the first chatbot is also illustrative of this.
Once a pioneer in the field, Weizenbaum (1976) soon becomes one of its deepest critics. Whilst ELIZA was modelled on the illusion of a Rogerian psychologist, he was horrified when he demonstrated it to psychotherapists to hear them actually considering that the model could be used to do their work.
For surely, he thought, in our darkest moments when we reach out to such professionals we are desperately seeking to be listened to by another human being, not simply engage with programmatic responses. (His fears are perhaps more chilling in today’s world of Cognitive Behavioural Therapy chatbots!)
AI systems then are mathematic models processes and run through computational logics, e.g., on ‘discrete stage machines’. And, AI development has been shaped through play, games and trickery (or illusion).
AI, Collective Memory and the Future
Our aim in attempting to disentangle AI pre-histories and histories from the anthropomorphic discourse which shapes public perception of these systems today was to then move forward with potential scenarios for thinking about how we might work with them in the future in ways that could make a productive contribution to collective memory. We suggested three possible scenarios:
Scenario 1: The Status Quo
Here, society refuses to move beyond anthropomorphism, thus the ‘simulative model prevails, i.e., we continue to invest in and work with AI models only with the aim of creating processes and outputs that seem to replicate what humans can do already. This approach has seen the reinforcement of hegemonic narratives. AI offers nothing new and we should ask, thus what’s the point in investing time, money and resource into it?
Scenario 2: Recognise AI’s Distinctiveness but Enfold it into Established Collections
In this case, AI is de-anthropomorphised to some extent but still considered to be a single entity or ‘being’ with agency equitable to humans, e.g., the ‘automation’. AI systems may help to empower marginalised voices, as discussed in work on Indigenous Epistemologies and AI, but it maintains a sense of ‘selfhood’ which downplays that they are fundamentally complex socio-cultural systems and are not already separate from humans. In this context, AI is used to support specific communities as they adopt their use to help amplify their voices, but does not necessarily open bridges between different groups.
Scenario 3: Complete De-anthropomorphisation of AI
Extending the relationality of scenario 2, this one – our ‘ideal’ – recognises AI’s radical computation alterity, yet also recognises ‘AI’ are already complex amalgamation of different systems incorporating the ‘human-in-the-loop’. In this respect, the agency sits with us as human communities and how we decide to unite and who we bring onboard to be represented in data sets, in training and supervision. This scenario opens up possibilities for tackling complex pasts, contested histories, and opening up cross-cultural dialogue through the development of new AI systems.
You can read the full, open access paper in Memory Studies Review via our publications page, or listen to Prof. Richardson-Walden’s recent presentation alongside other contributors to the special issue for the Memory Studies Association’s digital series dMSA here.