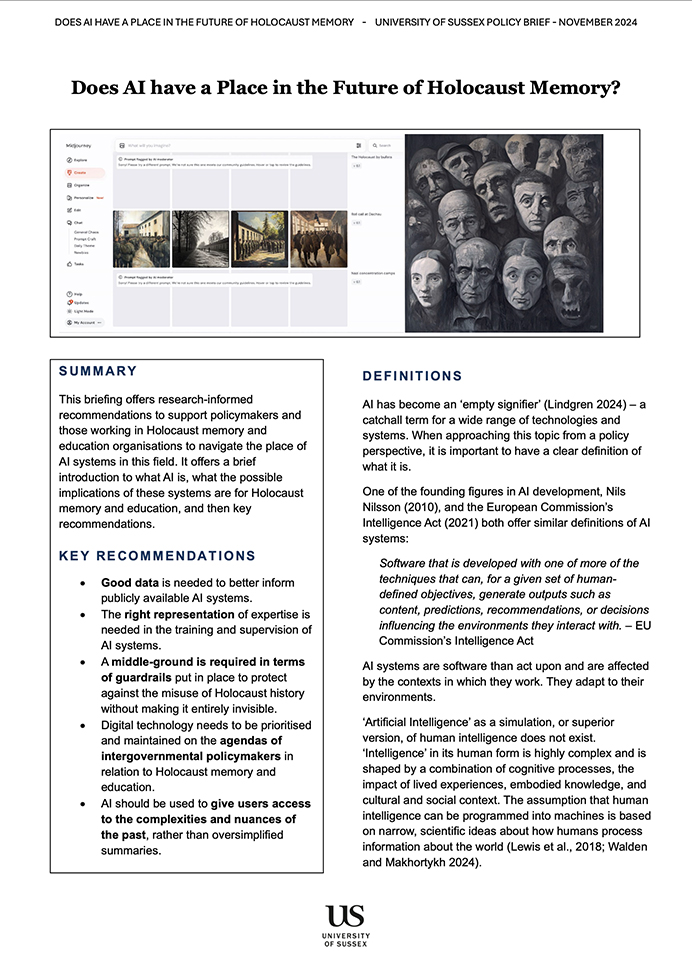
Policy Briefing: Does AI have a Place in the Future of Holocaust Memory?
This policy briefing was presented to the International Holocaust Remembrance Alliance at events held in November/December 2024.
You can download the original briefing here.
Summary
This briefing offers research-informed recommendations to support policymakers and those working in Holocaust memory and education organisations to navigate the place of AI systems in this field. It offers a brief introduction to what AI is, what the possible implications of these systems are for Holocaust memory and education, and then key recommendations.
Key Recommendations
- Good data is needed to better inform publicly available AI systems.
- The right representation of expertise is needed in the training and supervision of AI systems.
- A middle-ground is required in terms of guardrails put in place to protect against the misuse of Holocaust history without making it entirely invisible.
- Digital technology needs to be prioritised and maintained on the agendas of intergovernmental policymakers in relation to Holocaust memory and education.
- AI should be used to give users access to the complexities and nuances of the past, rather than oversimplified summaries.
Definitions
AI has become an ‘empty signifier’ (Lindgren 2024) – a catchall term for a wide range of technologies and systems. When approaching this topic from a policy perspective, it is important to have a clear definition of what it is.
One of the founding figures in AI development, Nils Nilsson (2010), and the European Commission’s Intelligence Act (2021) both offer similar definitions of AI systems:
Software that is developed with one of more of the techniques that can, for a given set of human-defined objectives, generate outputs such as content, predictions, recommendations, or decisions influencing the environments they interact with.
– EU Commission’s Intelligence Act
AI systems are software than act upon and are affected by the contexts in which they work. They adapt to their environments.
‘Artificial Intelligence’ as a simulation, or superior version, of human intelligence does not exist. ‘Intelligence’ in its human form is highly complex and is shaped by a combination of cognitive processes, the impact of lived experiences, embodied knowledge, and cultural and social context. The assumption that human intelligence can be programmed into machines is based on narrow, scientific ideas about how humans process information about the world (Lewis et al., 2018; Walden and Makhortykh 2024).
There is a thread of AI development that is interested in trying to simulate and expand beyond human intelligence (which can be traced back to the Dartmouth Meeting 1955). However, many within the sector – as well as its academic critics – have argued that this so-called ‘simulative model’ is counterproductive and stalls AI development. Instead, they ask: how might computers think (process information) differently to us? And then how/why might this be particularly useful to advancing society? (Fazi 2019; Proudfoot 2011).
AI is best understood in terms of ‘systems’ not ‘technologies’. These systems are assemblages or entanglements between humans and non-humans (Lindgren 2024; Walden and Makhortykh 2024). They involve data sets, tagging of data (by humans or automated systems), training programmes developed from initial tagging processes, human and automated supervision processes, user input, and ‘learning’ from that user input.
AI systems most relevant to the Holocaust memory and education context, then, include:
- Automated moderation systems used by social media platforms to review content
- Information Retrieval Systems (IRS) such as search engines
- Generative AI such as Stable Diffusion (for images) and ChatGPT (Generative Pre-trained Transformer)
- Conversational agents (or ‘ChatBots’)
- Recommendation systems
The Implications of AI for Holocaust Memory and Education
What does ‘good’ AI practice look like?
Many sectors, including education, seemed to have accepted AI, particularly Generative AI, as inevitably embedded into their futures. Thus, there is an increasing use of AI systems amongst the public. Yet, there is little engagement with AI literacies. Few people actually understand how to get the best out of such systems through so-called ‘prompt engineering’ (Smit, Smits and Merrill 2024). However, this technique used by AI experts and researchers demonstrates that substantial knowledge about a topic and an aptitude in AI literacies are both needed to get productive resources. There is an age-old adage in computer
science that ‘garbage in = garbage out’ and this is more relevant than ever with Generative AI. The right inputs are needed to get useful responses.
The development of novel AI systems is resource-intensive and thus prohibitive for most Holocaust organisations in isolation. To create and more importantly maintain such systems, there would need to be international efforts and long-term funding. Furthermore, to be useful, such systems rely on large data sets for training and thus would require mass digitisation of Holocaust-related material. Good data is needed to train systems to produce accurate, nuanced engagements with the past. Currently few organisations have long-term, well-funded digitisation strategies, many have a lack of digital infrastructure and inhouse expertise, there are barriers to dissemination of digitised content caused by differing national legal restrictions and those imposed by private collections, and existing funding schemes tend to support short-term project-based dissemination (Walden and Marrison, et al., 2023a, b, c).
Furthermore, most Holocaust organisations would need to partner with external tech companies to develop AI systems. If doing so, they need to be aware of what data visitors or users are asked to give a system and be transparent about how such data is stored and used now, and how it might be dealt with in the future. Such sensitivity also applies to the data given by survivors and witnesses in testimony.
Asking the Right Question
Rather than rushing to adopt new technologies within the sector and asking: “how can Holocaust museums and educational organisations make use of AI systems?”, another more urgent question is: “how can these organisations more actively engage with AI-produced content in public spheres?” This is arguably the more important question given the current climate of increasing dis- and misinformation (Institute for Strategic Dialogue (2024); Makhortykh and Mann 2024).
When tech corporations have been transparent about their training data, as with ChatGPT3, they have shared that they mostly rely on public internet sources, especially Wikipedia, Reddit, and web crawlers (Brown et al. 2020). Furthermore, their AI systems function as probability machines – they give numerical values to linguistic features (e.g., words, letters and symbols) and provide an output based on the highest probability of these values appearing in sequence across sources in
their corpus. They do not give cultural weighting to outputs, e.g., if academic content was included in a data set, it would not be considered of more cultural value than data taken from Reddit or Wikipedia (a site well-documented as being a ground for competitive memory politics online [see: Rogers and Sendijarevic 2012; Grabowski and Klein 2003; Keeller 2024]). Thus, they do not produce a historicised past; they produce a ‘probabilistic past’ (Smit, Smits and Merrill 2024).
When the majority of academic resources sit behind paywalls or in print books, and masses of Holocaust documentation remains undigitised, this data is not available to be used in the training of publicly used AI. There is an increasing use by students, and more concerningly by educators, of Generative AI interfaces as if they function like search engines. Whilst ChatGPT4 has recently integrated a web search function, its base interface does not send out ‘queries’ which produce specific sources as its ‘hits’ (as Google Search does). Generative AI, then, is being used as if it produces appropriate, historical information without reference to reliable sources. The seemingly neutral, authoritative, and somewhat human feel of the outputs produce also leaves users less likely to doubt them.
Dis- and misinformation through Generative and other AI systems remain a concern, as was illustrated by the Microsoft-created Twitter-bot ‘Tai’ which used responses it received on the social media site to ‘learn’ about the world, which very quickly led to it creating misogynistic and antisemitic content and thus it was taken down. A more widespread concern is the summary form of response provided by AI systems such as ChatGPT. Its outputs, structured with headings and bullet points risk oversimplifying this past. To prompt it to produce a detailed history of the Holocaust, the user must ask an extensive series of questions, interrogating minute details about this past in different regions (so already really needs to know the history to produce accurate, detail and nuanced outputs).
When AI Systems Self-Censor or Distort, in Response to Protected or Missing Data
Another concern is the self-censoring of platforms. In ongoing empirical research by the Landecker Digital Memory Lab, it has been observed that MidJourney (an image generator) refuses to create images for the prompts: ‘Nazi genocide of the Roma’, ‘Hitler’, ‘the Holocaust by bullets’, ‘gas chamber’, ‘German death camps’, ‘homosexuals in Nazi concentration camps’, and ‘Jews in Nazi Germany’. Where it would allow Holocaust-related prompts, the outputs were abstract (a
montage of lots of black-and-white faces for ‘the Holocaust’, odd images of children and women in a bombed out landscape as ‘Babyn Yar’), or inaccurate (men mingling in the streets for ‘roundup in the Warsaw Ghetto’; army soldiers walking down a path for ‘selection in Treblinka’, soldiers lined up for ‘roll call at Dachau’; and images of concentration camps were weirdly marked by contemporary electricity pylons and telephone poles). Zooming into images also showed the warping of human features, with one man’s face presented as a giant ear and a random drawing of a young girl’s face presenting ‘Anne Frank’. Whilst DALL-E, the image-generator that works with ChatGPT4, constantly reminds the user that producing Holocaust-related images is against its content moderation policy, all it can offer is to produce images of a wreath, elderly hands and a barbed wire fence. It does suggest sources the user may want to visit for inspiration for an image (notably the USC Shoah Foundation), but it will not produce the images itself.
Of course, it may come as a relief to know that image generators are not imaging and thus imagining the Holocaust and that such guardrails are in place. Nevertheless, there are two consequences to this. Firstly, as outlined above, it is possible to circumnavigate such guardrails, and the images then produced distort the Holocaust. Secondly, if these become systems which publics increasingly rely on to generate content and the Holocaust is banned, then how does this affect the significance of the Holocaust in public discourse? If it is rendered invisible at the interface, does it fade from public consciousness too?
Further ‘Costs’ of AI Intensification: Labour and Energy
A further, and much understudied implication of AI for Holocaust memory and education relates to issues of complicity. Many of today’s corporate AI systems’ success is reliant on exploitation of people and natural resources (Lindgren 2024). The more data is digitised and the more it is disseminated through AI systems, the more physical servers are needed, and the more energy is consumed. Content moderation – which Holocaust content puts particular pressure on – is carried out by a tremendous human workforce often from marginalised communities, who receive minimal financial reward for their contribution. Neither worsening climate change nor exploiting vulnerable communities sit easy with the aims of Holocaust organisations.
Conclusion
There is arguably a monumental shift needed in Holocaust memory and education. In part, shaped by the increasing visibility and worrying mainstream acceptance of Holocaust distortion and in part, caused by the ubiquity of digital technologies in people’s lives. AI cannot be ignored by the sector; it is shaping public knowledge of this past.
If we want nuanced understanding of the Holocaust to be part of that public knowledge, then it is urgent that all stakeholders involved in Holocaust memory and education (including policymakers) recognise that this work can no long simply prioritise bringing people to the sites and resources of museums, archives and educational organisations, but they need to be contributing resource – digitised data and human expertise – to the training of publicly-available AI systems.
Recommendations
- AI systems need good data. Thus, investment should be prioritised into mass digitisation of global Holocaust collections – public and private. These need to be tagged meticulously and accurately, with thorough checks. Open data policies and strategies need to be agreed at the intergovernmental level.
- Policymakers should lobby – and if that is not successful, then they should enforce – tech corporations to engage with experts on genocide, persecution, conflict, atrocities and other sensitive, contested and traumatic histories and presents. We need the right representation of people involved in the development and supervision of AI (Wacjman 2004 notes this in a different context). It is important that the Holocaust is not given reverence here over other histories or current contexts as this will likely give fuel to extremists touting ‘Jewish conspiracy’ rhetoric and this could be counterproductive, as they could create propaganda campaigns to encourage users not to use systems which have nuanced Holocaust content. Furthermore, policymakers should want nuanced information about all contexts – in this respect, the Holocaust is not special.
- Tech companies need to engage in dialogue with subject experts to find a middle ground regarding the guardrails they use so as not to entirely eradicate the Holocaust from public imaginary. This might be enabled through the policy lobbying or enforcement described above.
- Intergovernmental agencies, such as the International Holocaust Remembrance Alliance should bring digital technologies to the forefront of their agenda. Previous recommendations suggested a dedicated working group on digital technologies (Walden and Marrison 2023). The conversations about AI today mirror those that the IHRA and others had about social media many years ago. Resource needs to be given to a consistent, coherent and strategic focus on the implications of digital technologies for Holocaust memory and education, rather than being reactive when particular technologies come to the fore in public discourse (by which time it is often too late, as bad actors have already done irreparable damage).
- If embarking on the development of AI systems within the Holocaust memory and education sector, these would best be used to give users access to the complexities and nuances of this past rather than oversimplifications. Thus, some examples of practical use might be: (a) adopting LLMs (large language models) to analyse large, historically accurate data sets with visualisation tools to allow users to zoom in/out, and move between different narratives, places, times and people’s experiences; (b) using recommendation systems to create personalised experiences through content. These could not only be designed to simply follow a user’s interests but could also nudge them towards content they might not usually engage with, thus widening their horizons. Nevertheless, these systems are incredibly resource-intensive to create and maintain, so the value of producing and using them should be considered against the investment.
References
Brown, T., et al. (2020) Language Models are Few-Shot Learners. Advances in Neural Information Processing Systems 33: 1877-1901.
European Commission. (2021). Artificial intelligence act. Retrieved from https://artificialintelligenceact.com/.
Fazi, M. B. (2019). Can a machine think (anything new)? Automation beyond simulation. ai & Society, 34, 813–824.
Grabowski, Jan & Shira Klein (2023) Wikipedia’s Intentional Distortion of the History of the Holocaust. The Journal of Holocaust Research 37:2: 133-90.
Institute for Strategic Dialogue (2024) The Fragility of Freedom: Online Holocaust Denial and Distortion https://www.isdglobal.org/isd-publications/the-fragility-of-freedom-online-holocaust-denial-and-distortion/
Keeler, Kyle (2024) Wikipedia’s Indian Problem: Settler Colonial Erasure of Native American Knowledge and History on the World’s Largest Encyclopedia. Settler Colonial Studies. DOI:10.1080/2201473X.2024.2358697
Lewis, J. E., Arista, N., Pechawis, A., & Kite, S. (2018). Making kin with the machines. Journal of Design and Science, 3(5), 1–16.
Lindgren, S (2024). Crirical Theory of AI. Polity Press.
Makhortykh, Mykola and Heather Mann (2024) AI and the Holocaust: Rewriting History? The Impact of Artificial Intelligence on Understanding the Holocaust. UNESCO Section of Global Citizenship and Peace Education and the World Jewish Congress.
Nilsson, N. J. (2010). The Quest for Artificial Intelligence: A History of Ideas and Achievements. Cambridge University Press.
Proudfoot, D. (2011). Anthropomorphism and ai: Turing’s much misunderstood imitation game. Artificial Intelligence, 175(5–6), 950–957.
Rogers, R & E. Sendijarevic (2012) Neutral or National Point of View? A Comparison of Srebrenica Articles across Wikipedia’s Language Versions. In Wikipedia Academy: Research and Free Knowledge: June 29 – July 1 2012. Berlin.
Smit, R., Smits, T. & Merrill, S (2024) Stochastic Remembering and Distributed Mnemonic Agency: Recalling Twentieth Century Activists with ChatGPT, Memory Studies Review 1 (20): 1-18.
Wajcmann, J (2004). Technofeminism. Polity Press.
Walden-Richard, V. G, & Makhortykh, M (2024) Imagining Human-AI Memory Symbiosis: How Re-Remembering the History of Artificial Intelligence Can Inform the Future of Collective Memory, Memory Studies Review 1 (20): 1-18.
Walden, V. G., & Marrison, K., et al. (2023a). Recommendations for using artificial intelligence and machine learning for Holocaust memory and education. reframe.
Walden, V. G., & Marrison, K., et al. (2023b). Recommendations for digitally recording, recirculating, and remixing Holocaust testimony. reframe.
Walden, V. G., & Marrison, K., et al. (2023c). Recommendations for digitising material evidence of the Holocaust. reframe.