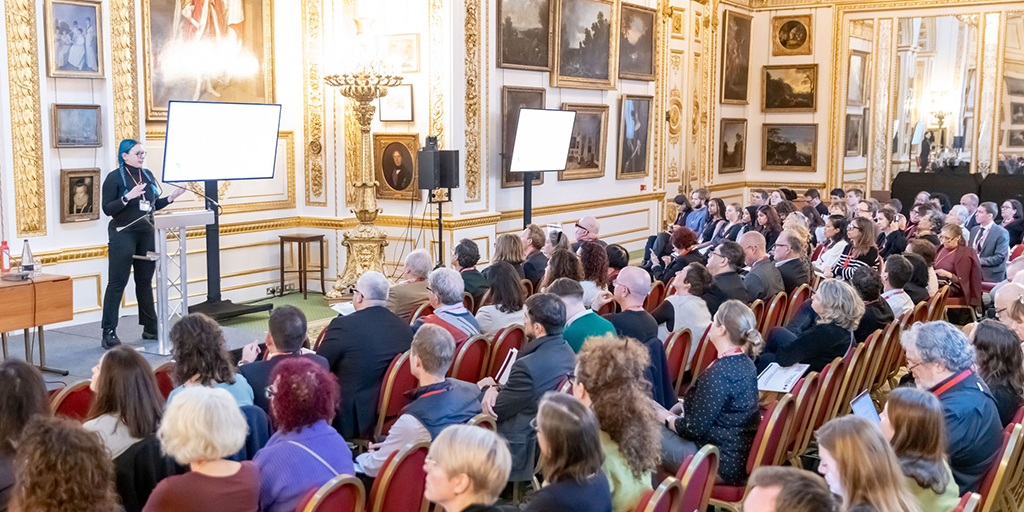
10 key implications for AI in Holocaust memory and education
by Victoria Grace Richardson-Walden
Our Lab Director has recently been engaging with delegates of the International Holocaust Remembrance Alliance about AI and Holocaust memory, tackling topics including mass digitisation of archival and historical material and the risks of distortion and disinformation. In this week’s blog she discusses what emerged from recent events organised by the IHRA, including our new policy briefing.
I have had the pleasure to engage with the International Holocaust Remembrance Alliance (the IHRA) on two occasions in the last few weeks because under their UK Presidency in 2024, it has decided to focus on the significance of AI for Holocaust memory and education.
Firstly, I spoke at an online AI workshop with approximately 70 people, organised by the IHRA’s Education Working Group. Then, this past weekend, I presented the opening paper at the conference ‘AI in the Holocaust Education, Research and Remembrance Sector’ at Lancaster House, London.
My role at these events was really to set the scene for those who are policymakers and Holocaust education and history experts, but less savvy about emerging digital technologies.
At the core of my presentations was the question: what are the implications of AI for Holocaust memory and education in light of the rising popularity of Generative AI models and deep fakes?
These, I argued, are 10-fold.
Domain-specific AI development is resource intensive
Large Language Models (LLMs) – which seem to be of particular concern to the IHRA – are incredibly resource intensive. Currently, the most prominent example of the use of AI, or at least, machine learning in Holocaust memory is the USC Shoah Foundation’s Dimensions in Testimony project.
This uses Natural Language Processing, however it is not, in the wider context of AI development, computationally complex. It is a heavily supervised model and relies on substantial human intervention throughout its continuous training. It would be better described as a Small Language Model or a series of Small Language Models.
Given the USC Shoah Foundation is much larger and much better staffed with digital expertise than most Holocaust memory and education organisations, it is unlikely that individual institutions or even national, regional or international collaboration can support the financial, human and computational resource needed to develop complex LLMs (which process trillions of parameters in seconds).
Thus, it might be better to direct the sector’s expertise towards informing commercial models and to focus on the mass digitisation of Holocaust-related data so that it is made available for scraping. In other words, inform these models with accurate information.
We know that ChatGPT3 was heavily reliant on Wikipedia and Reddit for its content. Do we not want these publicly-used models to be more reliant on historical nuance rather than spaces where expertise does not count?
At the same time, we must be vigilant to the corporate motivations of the organisations who create these commercial models and the malicious business practices of some.
Garbage in = garbage out
We need the right data and expertise to inform models in the first place. This emphasises the need for Holocaust experts to work in partnership with those creating Generative AI models and for policymakers and funders to support mass digitisation of Holocaust archival materials and historical texts.
Users also need to know how to ask the right prompts to get the answers they want, otherwise their input produces useful output.
Users need the right expertise
AI models that generate ‘answers’ to queries can be useful for testing one’s existing knowledge or refining ideas. However, our research has demonstrated that you need to know a lot about a subject already – at least in relation to the Holocaust – to both (a) get useful outputs and (b) have the confidence that they are correct.
We see this for example when we ask ChatGPT4 to explain the Holocaust for high school students. It gives a very brief summary and a general history focusing solely on the Nazis as perpetrators and uses phrases like the ‘darkest chapter in history’. When we ask it to give details of ‘lesser-known narratives of the Holocaust’, it provides topics already presented in most Holocaust museums, from partisan groups to the Kindertransport. We have to specifically ask about lesser-known narratives, such as the Occupation of the Channel Islands and the Holocaust in North Africa, for it to populate any facts about these topics.
Generative AI models are ‘probability machines’ not ‘knowledge machines’
Generative AI, like ChatGPT4, are mathematical models. None of them ‘understand’ or give meaning or cultural value to the linguistic signifiers with which they work, i.e. words, sentences and symbols.
Instead, these assets are given ‘numerical values’ and are processed by the models as ‘tokens’. The models then search across their available data corpus to find existing patterns in which these ‘tokens’ exist and produce an output based on the most probable answer of other tokens that follow.
Generative AI models reproduce canons and value virality over historical nuance
This probability logic means you’re more likely to (re)produce well-known, ‘canonical’ outputs, for example, the Holocaust = Auschwitz, rather than lesser-known stories which get pushed even further to the periphery.
In our research on image generator ‘MidJourney’, we see that most Holocaust-related images that it will produce (and it tends to censor more than produce), look a bit like Auschwitz.
A reliance on the existing practice in AI development risks the work the IHRA has done in preserving at-risk sites and on increasing public knowledge about the Holocaust by Bullets (just two examples), being forgotten.
More to the point, the ‘canon’ is already well-established and in online public discourse (the type of content mostly scraped), it is the simplistic, ‘grand narratives’, major sites, and famous stories and people that circulate the most, for example, ‘Hitler’ and ‘Anne Frank’.
Information is given value in these systems based on its circulation or virality, rather than its historical truth or nuance.
ChatGPT3 was heavily reliant on Wikipedia and Reddit. Do we not want these publicly-used models to be reliant on historical nuance rather than spaces where expertise does not count?
Are summaries enough?
Commercial LLMs produce simplified, bullet-point ‘briefs’ on a topic. They summarise and with this, lose the necessary nuance needed in exploring the complex histories of the Holocaust.
Do we not want nuance and complexity to be at the fore in Holocaust education and memory?
It is important to recognise that it becomes far easier for bad actors to instrumentalise the Holocaust for political means if the majority of the public only have a simplified understanding of this past.
We need to enhance digital literacies
Anyone engaging with AI, including users, teachers, cultural organisations and policymakers, needs a fundamental understanding of how it works and the implications of using it before relying on AI for the production of knowledge.
And let us remember: AI models are not ‘knowledge machines’ yet so many people use them as such.
I recently wrote an article for Teaching English magazine on AI literacies, in which I explained that a good programme would focus on:
- An understanding of the workings of AI models
- Knowing the right things to ask
- Developing critical thinking skills to assess outputs
- Activities that attempt to design AI considering the ground truths needed to inform models
- Learning at least basic code
Beware the hype – think about long-term digital strategy
A warning I gave at the beginning of my IHRA conference presentation is I think fundamentally important. AI is the current buzzword that the tech industry, its PR agents and hedge funders want us to focus on and they’re pretty persuasive.
The IHRA and many organisations have a habit of being reactive when particular digital media come into the spotlight. This happened previously with social media. However, this is counter-productive. A far better approach would be to adopt a long-term digital strategy, which constantly considers the different digital developments affecting the sector.
The IHRA to-date does not have a working group or a long-term committee or focus on digital media and technologies. Yet delegates and many across the broader global Holocaust memory and education sector are very aware of the increasing visibility of Holocaust denial and distortion through digital platforms.
Current guardrails are not sufficient
Our research has demonstrated that there are – perhaps surprisingly – strong guardrails in place on many Generative AI models. However, we argue that these are not fit for purpose and may actually harm the development of Holocaust memory and education rather than defend against denial and distortion.
Firstly, it is very easy to circumnavigate such guardrails as one speaker at the AI conference explained in relation to Holocaust distortion online. Secondly, there is the danger that if these models become increasingly used by the general public as the go-to place for information but simple prompts like ‘Nazi concentration camp’ are censored (as we discovered on MidJourney), this has the potential to render this past invisible in a culture increasingly reliant on visual media.
Recognising our role in current-day exploitation
It is so important and so often overlooked that commercial AI models exploit vulnerable communities – those employed on poor wages, mostly in central Africa and in parts of Latin America to tag data and moderate content.
The Holocaust is one of the most highly moderated topics, so everything we create with these systems related to this past adds to the exploitative labour of these individuals.
These models also exploit our natural resources from the mining of lithium to huge data server centres, and the masses of power they use. This is counter to our drive towards meeting global sustainability goals.
Neither of these more hidden outputs sit comfortably with the human rights agendas of Holocaust memory and education organisations. Indeed, after having shared this warning, it was disappointing to see colleagues throughout the conference just ‘ask ChatGPT’ for some content to share as if this was a bit of a ‘joke’.
Alongside my presentation, the IHRA conference included a broad programme including how extremists use AI to generate antisemitic content, from Danny Morris (Community Security Trust), a panel on technology, memory and ethics, including academics Dr Rik Smit and Sam Merrill, and Pedagogical Director of Yad Vashem, Dr Yael Richler Friedman.
There were also presentations from Noah Kravitz (NVIDIA AI Podcast), Dr Robert Williams (USC Shoah Foundation), Shiran Mlamdovsky Somech (Generative AI for Good) and Clementine Smith (Holocaust Educational Trust).
Please do get in touch with the Lab if you would like to talk further about responsible use of AI for the sake of Holocaust memory and education.
What to Know More?
Read the full policy briefing here
Hear what our recent visiting fellows have to say on the topic of AI and Holocaust memory